The Change You Want To Detect
Semantic Change Detection In Earth Observation With Hybrid Data Generation
LASTIG(1) - IGN(2) - ENSG(3)
(2) : Institut national de l'information géographique et forestière / French national mapping agency
(3) : École nationale des sciences géographiques
Semantic Change Detection (SCD) in remote sensing comes with its fair share of challenges. Especially when tackling the task on very high resolution pairs of aerial images. Pixel-level annotated Semantic Change datasets as HRSCD [(missing reference)], SECOND [(missing reference)] or HiUCD [(missing reference)] exists but each comes with its drawbacks. Lack of diversity, scarce and coarse annotations, limited resolution, etc.
Training large deep learning models on these data is possible, but doesn’t offer any generalization capacity. Annotating large set of data would require giant amount of time. In such a context, simulation and especially synthetic data generation appears as a really good solution to mitigate these difficulties.
FSC-180k : A large-scale hybrid dataset for very high resolution semantic change detection
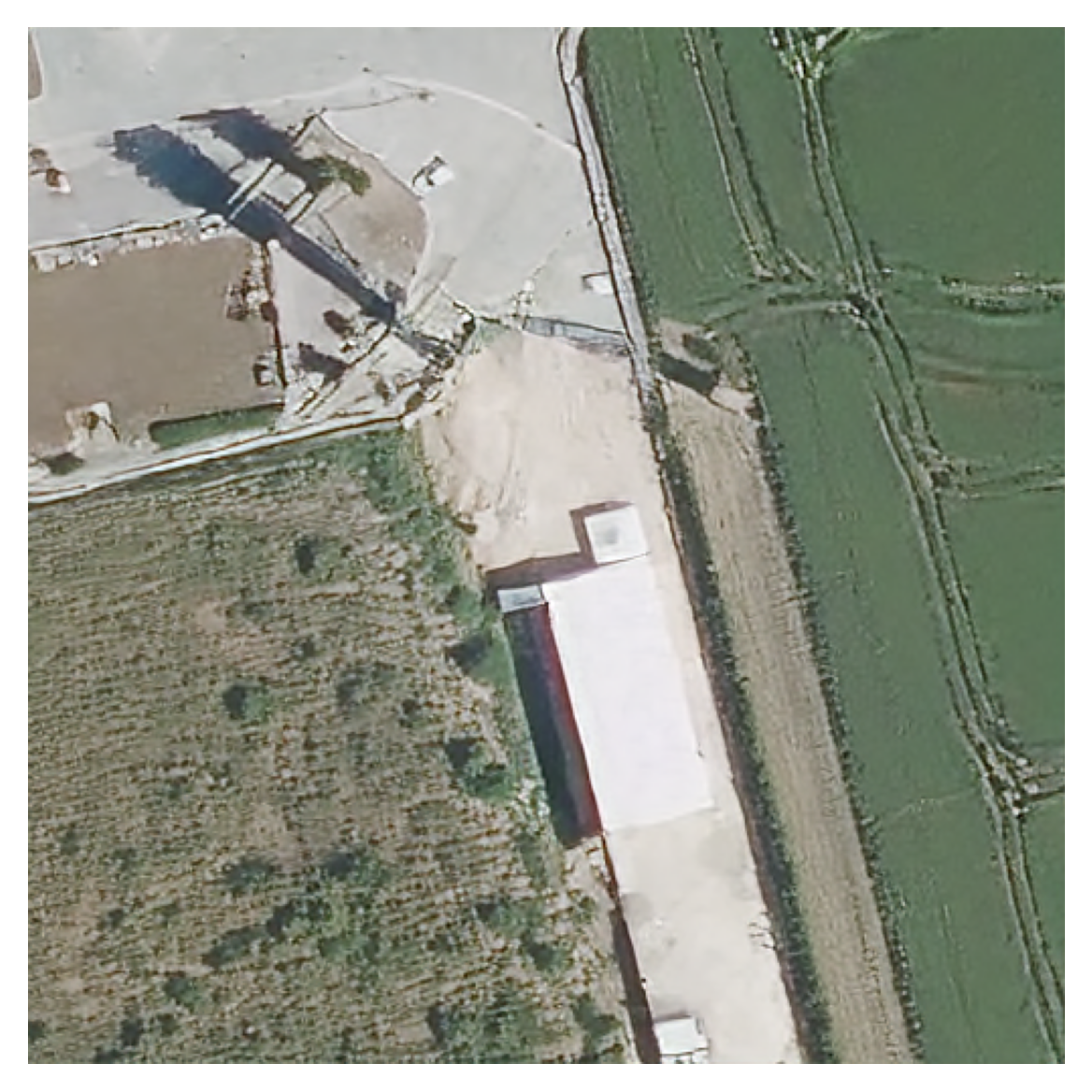
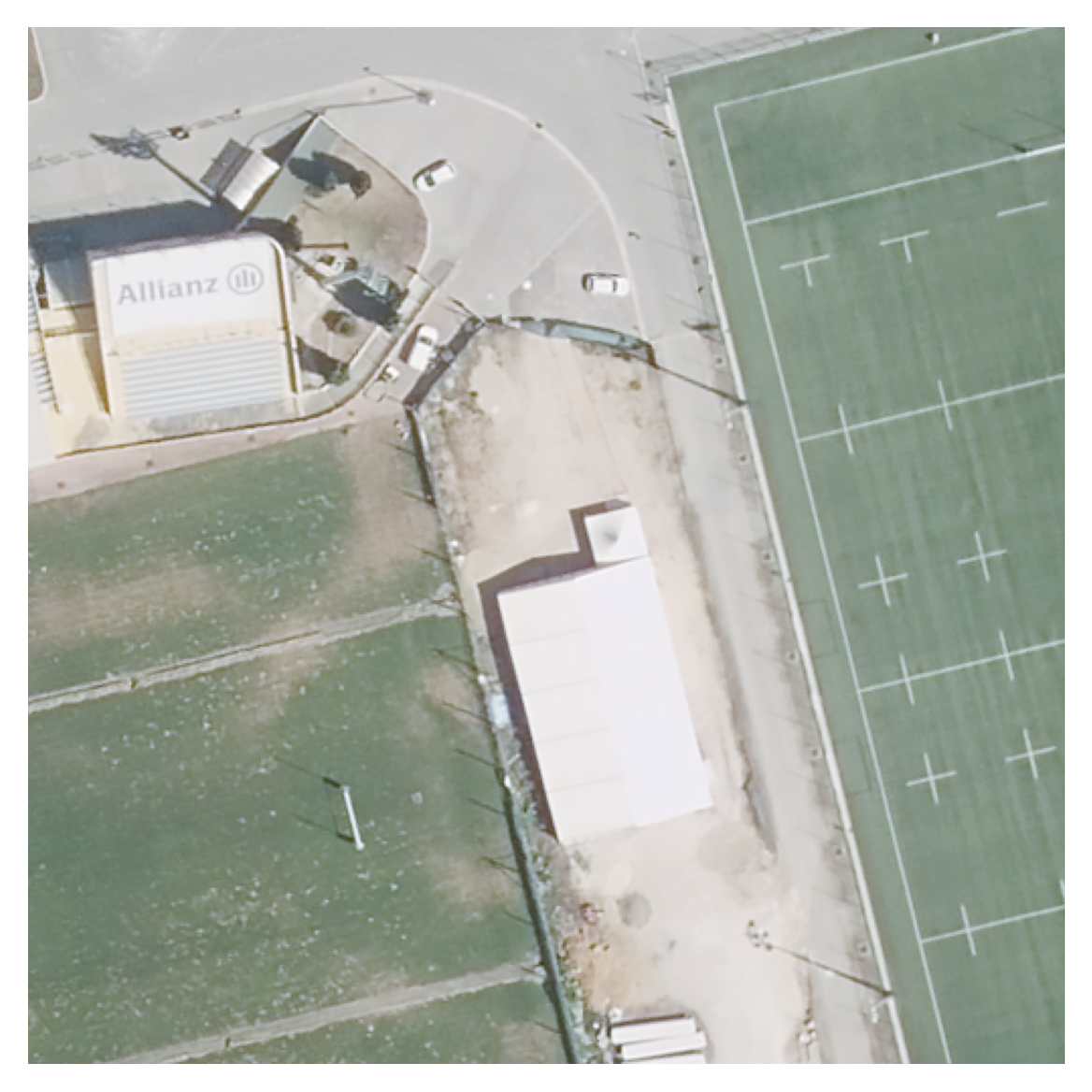
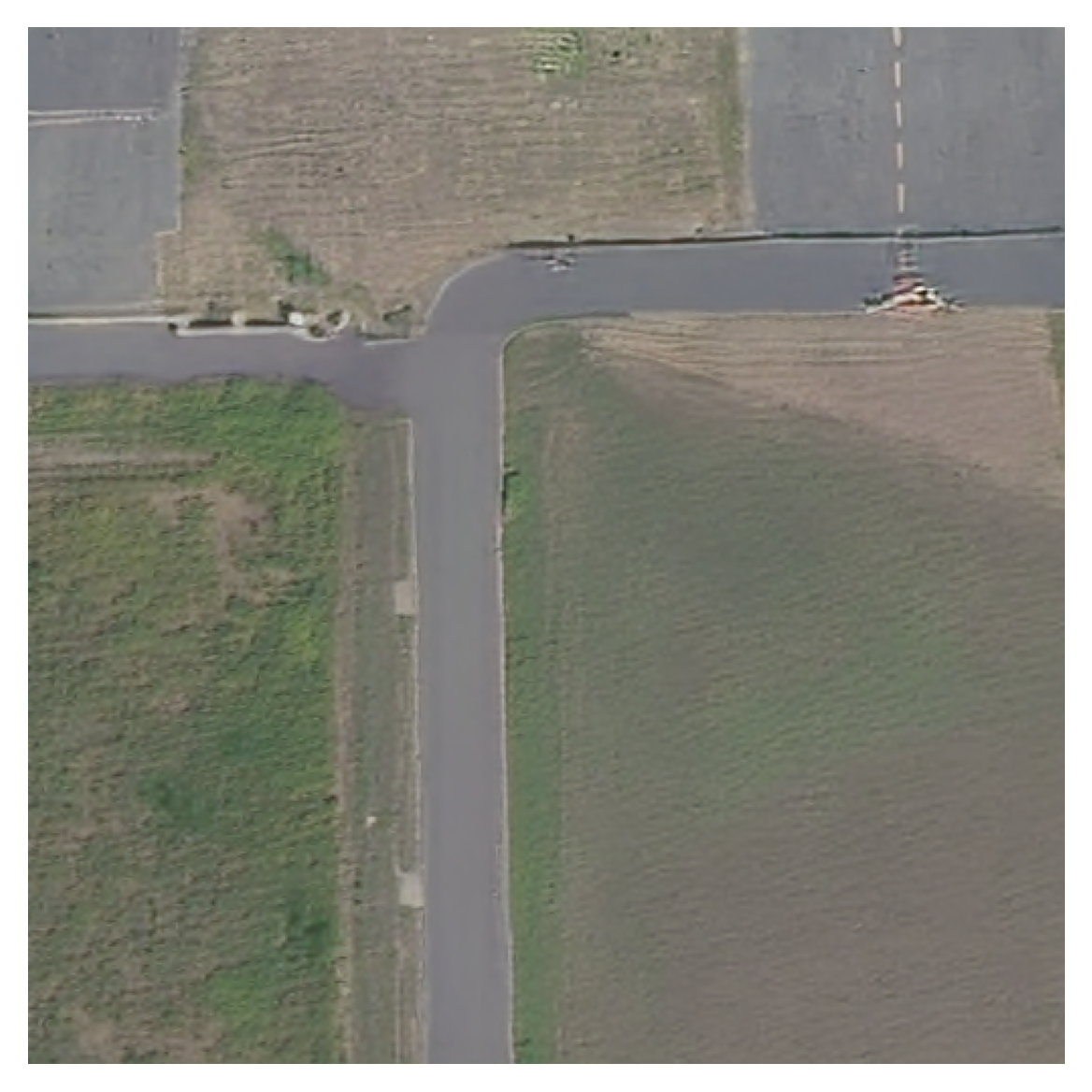
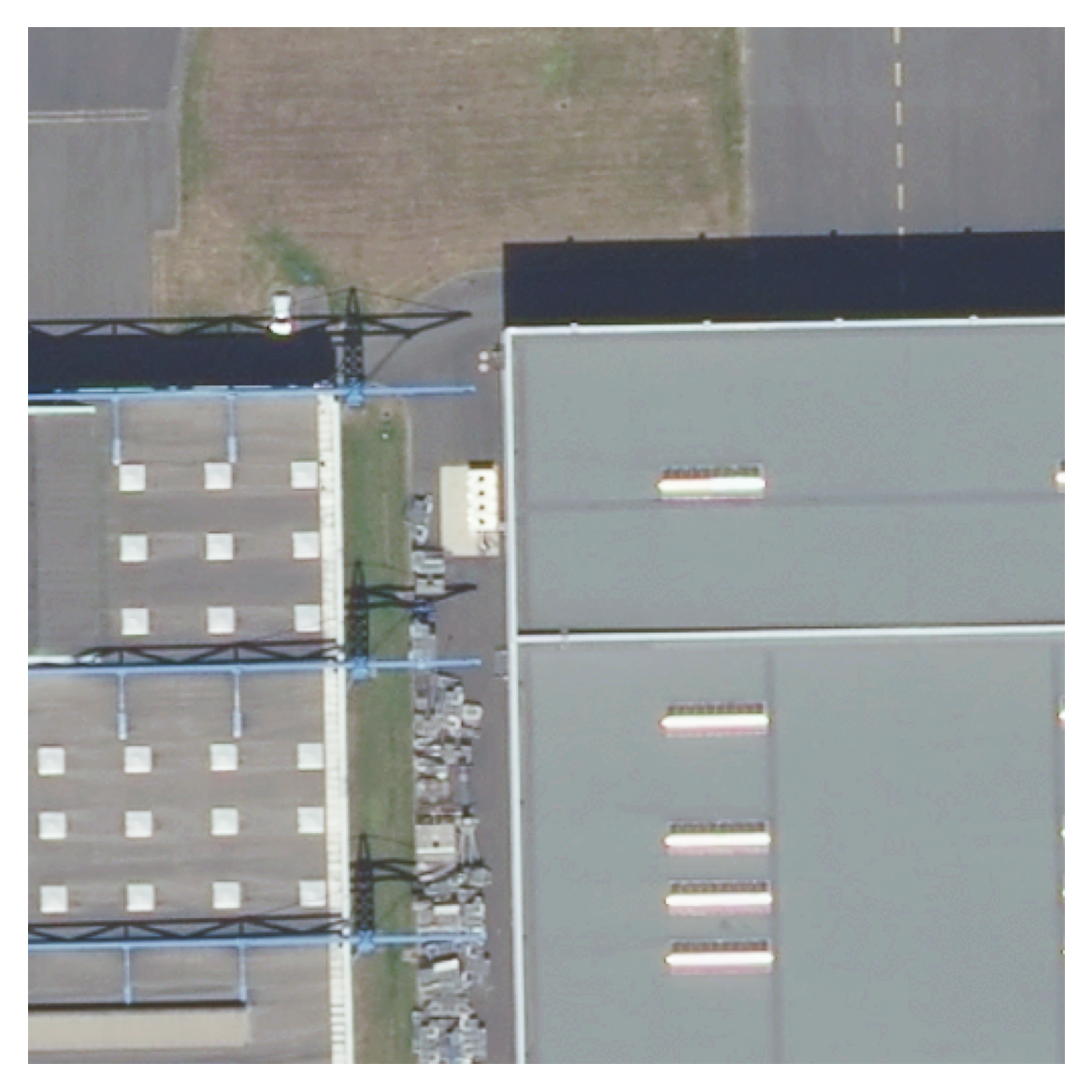
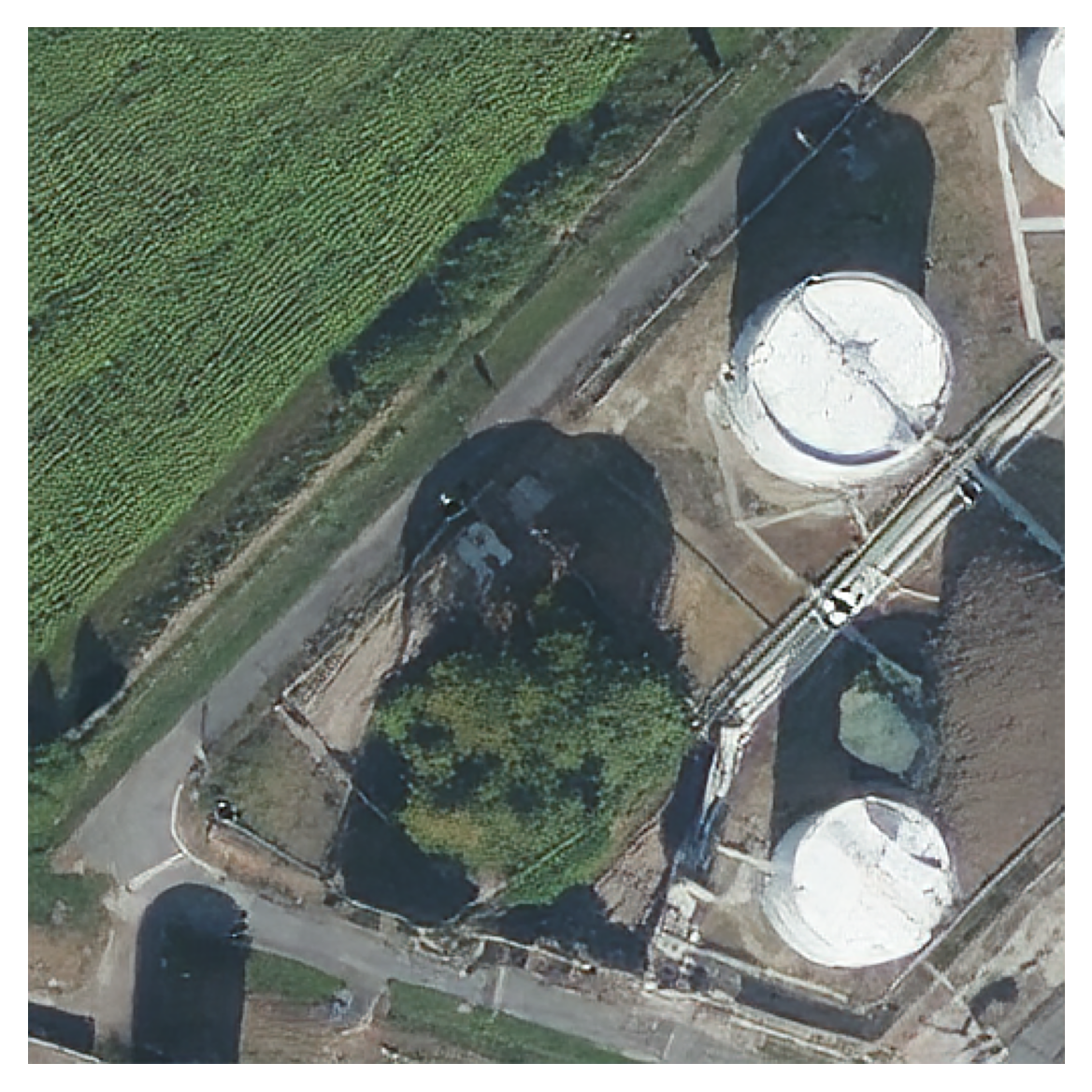
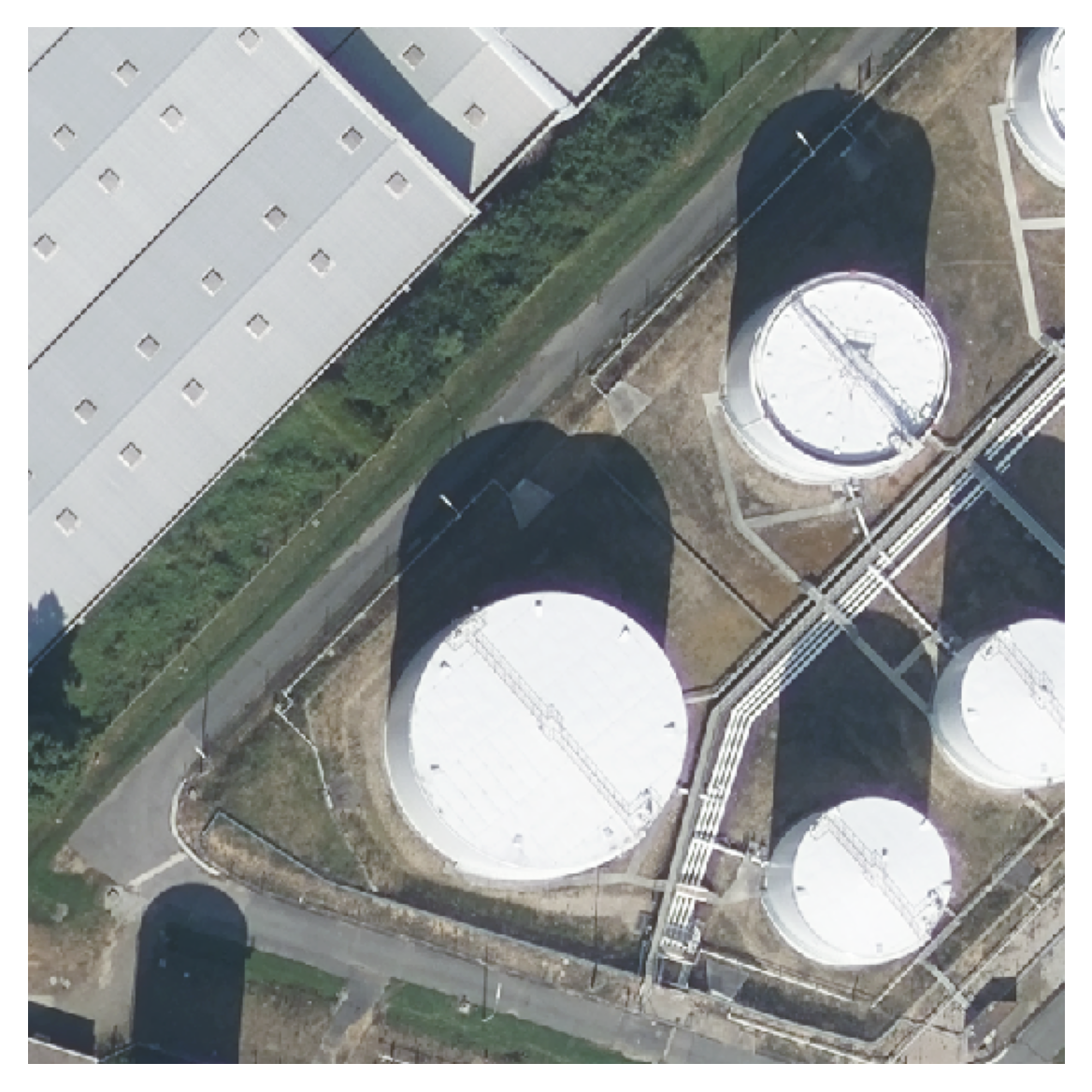
What we propose : hybrid data generation and efficient transfer learning
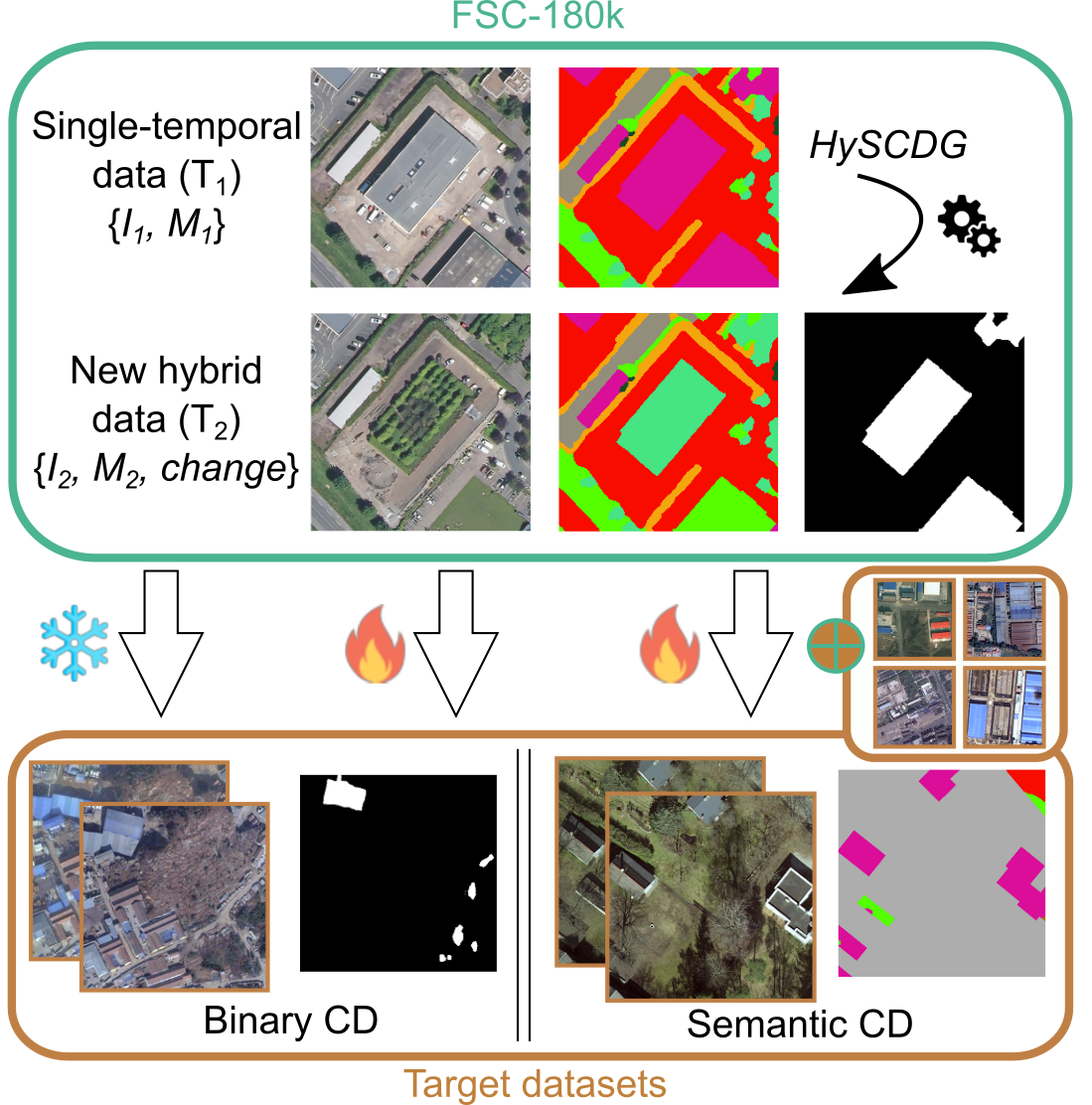
Generating changes ? How it works ?
Leveraging Stable Diffusion and ControlNet through long training on VHR aerial images, we create an end-to-end “change inpainting” pipeline. Diffusion models allow to produce realistic and various textures to fulfill the original image while the control module is responsible for monitoring the semantic composition of the generated image.
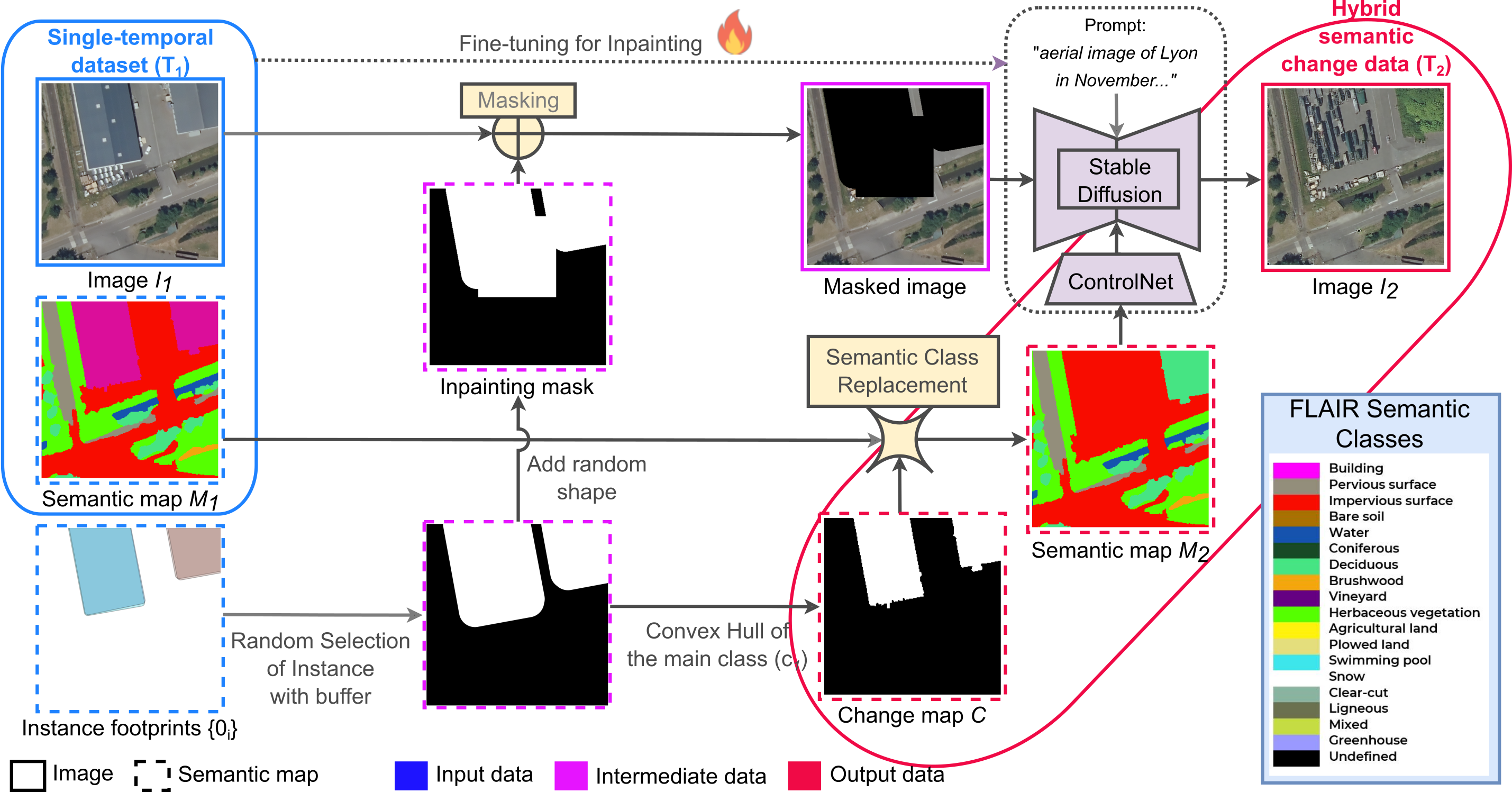
Transfer learning to enhance real use-cases change detection
We assess the quality of our hybrid dataset by using it in 4 transfer learning schemes : sequential, mixed, low data regime and zero-shot. Evaluation is done on 5 different real target change detection datasets. In all cases, the performance is improved thanks to the pre-training, proof of the contribution and versatility of FSC-180k. We outperform scores obtained by using an other synthetic dataset, SyntheWorld.
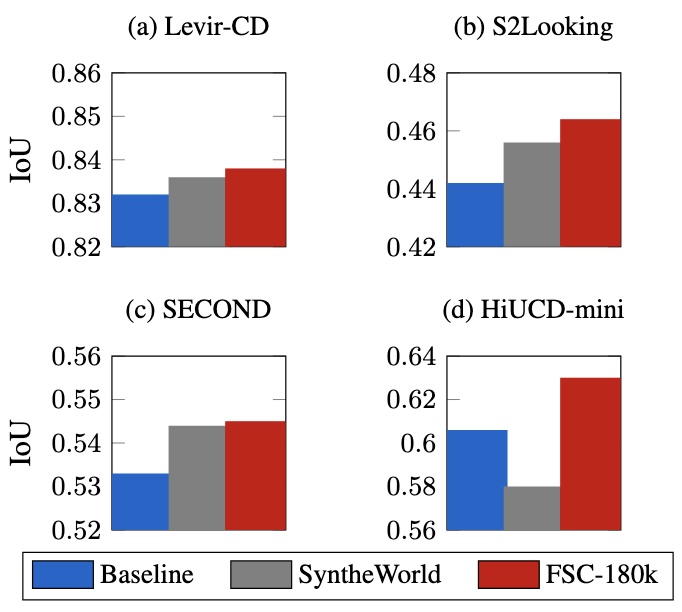
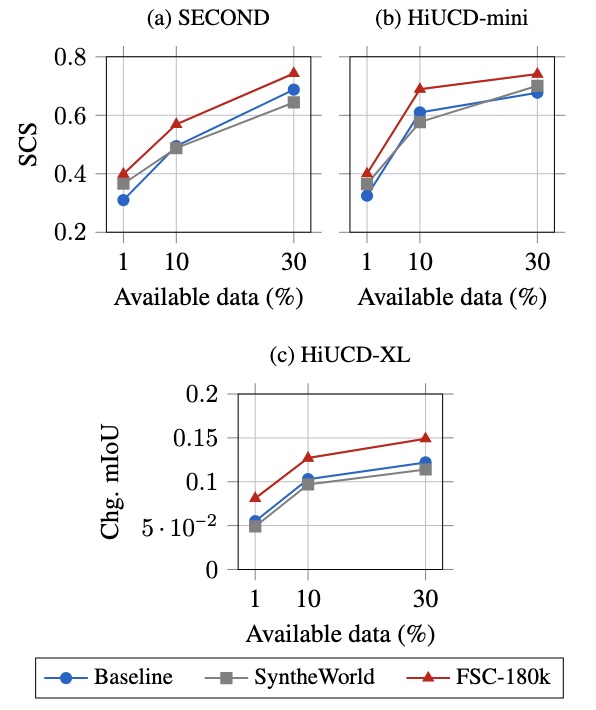
And visually...
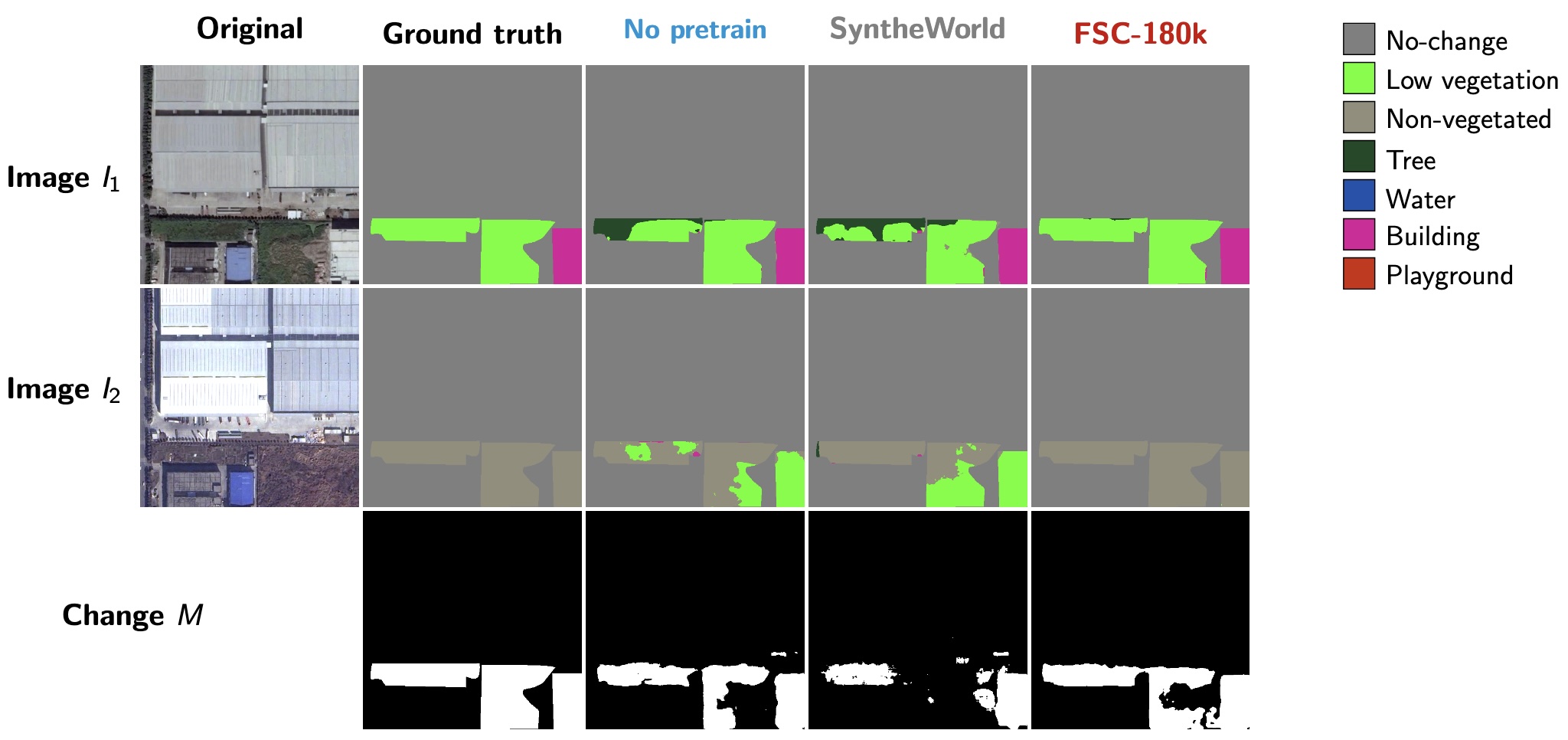